The Extraordinary Evolution of Training Data for Self Driving Cars
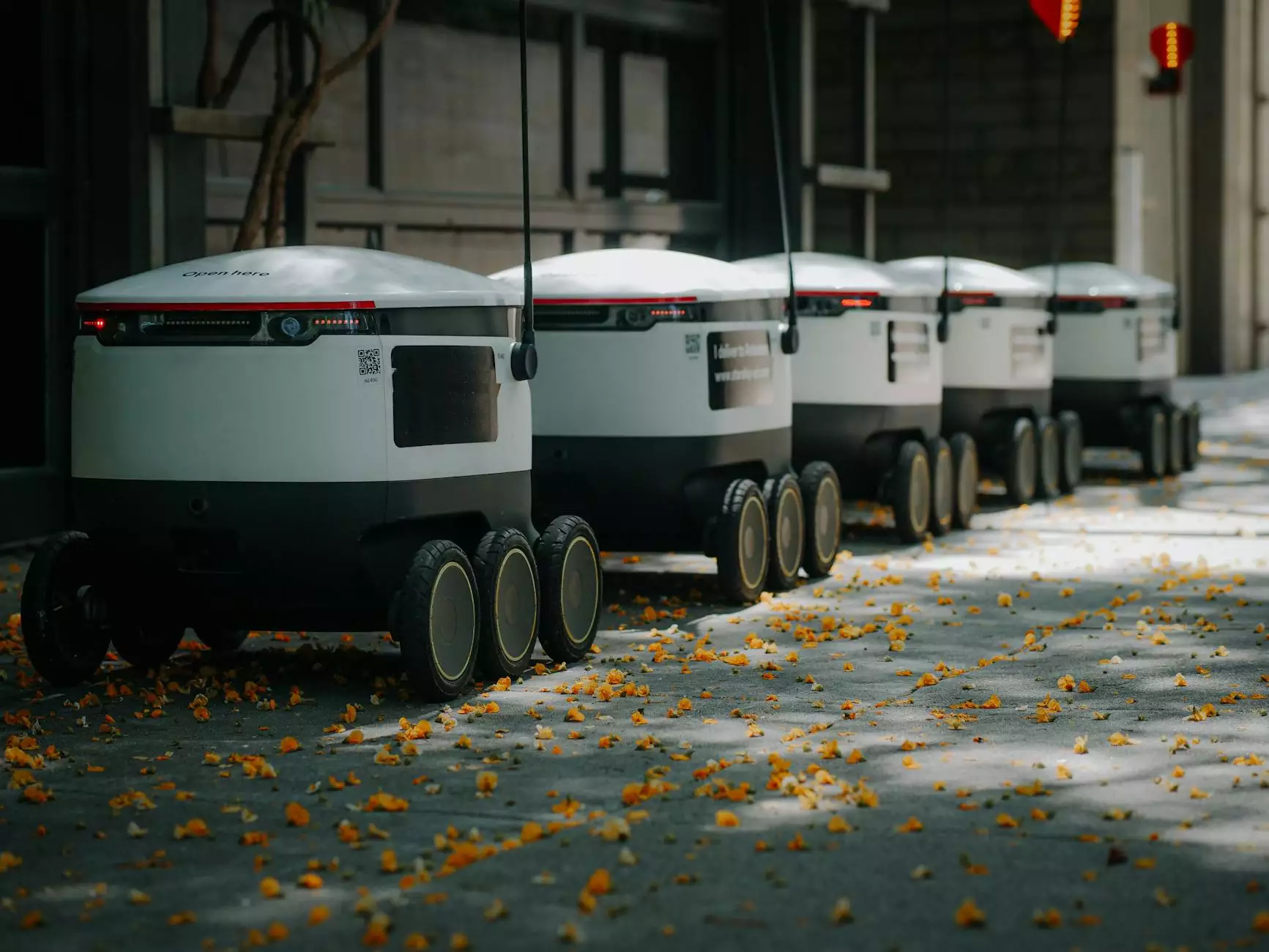
Self-driving cars are no longer a concept confined to science fiction. Instead, they represent the culmination of advanced technology, sophisticated algorithms, and a vast array of data—specifically, training data for self driving cars. This article dives into the fundamental aspects of this critical element driving the automotive revolution.
The Significance of Training Data
In the realm of artificial intelligence (AI) and machine learning (ML), training data acts as the bedrock upon which predictive algorithms are built. For self-driving vehicles, quality training data is essential to ensure safety, efficiency, and reliability. Here are several reasons why training data is a vital asset for autonomous vehicles:
- Vehicle Behavior Patterns: Training data helps in modeling how vehicles should behave in varying situations, such as merging into traffic, stopping at red lights, or avoiding obstacles.
- Environmental Understanding: Data collected from different environments, such as urban areas, highways, and rural settings, allows self-driving cars to comprehend and navigate diverse landscapes.
- Safety Enhancements: Quality training data can minimize accident risks by teaching vehicles to recognize potential hazards and make informed decisions.
- Prediction Capabilities: Predictive models based on training data allow autonomous systems to anticipate the actions of other drivers, pedestrians, and cyclists.
Types of Training Data for Self Driving Cars
Training data is not homogenous; it can be categorized into several types, each playing a significant role in the autonomous driving process:
1. Sensor Data
Self-driving cars are equipped with a multitude of sensors, including cameras, LiDAR, radar, and ultrasonic sensors. The data from these sensors is critical for:
- Object Detection: Identifying other vehicles, pedestrians, traffic signs, and obstacles.
- Distance Measurement: Accurately gauging the distance to other objects on the road.
- Environmental Mapping: Constructing a real-time map of the vehicle's surroundings.
2. Labelled Datasets
Labelled datasets are curated collections of images and data where each element is tagged with the appropriate classification (e.g., cars, pedestrians). This type of data is vital for:
- Deep Learning Models: Training neural networks to recognize and categorize objects effectively.
- Algorithm Improvement: Providing clear examples helps refine algorithms to reduce false positives and negatives.
3. Simulation Data
With advancements in technology, simulated environments allow for the generation of synthetic data, which is instrumental in:
- Diverse Scenarios: Creating a wide variety of driving scenarios, some of which may be rare in the real world.
- Safety Testing: Conducting tests in controlled environments without the risks involved in real-world testing.
Generating Quality Training Data
The generation of effective training data is both an art and a science. It requires collaboration among various disciplines, including data science, engineering, and domain expertise. Here are key strategies for generating high-quality training data:
- Data Collection: Involves gathering raw data from different sources and environments using vehicles equipped with advanced sensing technologies.
- Data Annotation: Carefully tagging and organizing data to ensure it is usable for training models.
- Data Augmentation: Enhancing datasets by creating variations of existing examples, thus increasing the robustness and diversity of the training set.
The Challenges of Working with Training Data
While generating training data is crucial, it is not without its challenges. Some common issues include:
- Data Quality: Ensuring that the data is accurate, relevant, and reflective of real-world situations.
- Data Bias: Addressing potential bias in datasets that could lead to biased decision-making by autonomous systems.
- Legal and Ethical Considerations: Navigating regulations related to data privacy and the ethical implications of using certain data types.
The Role of Machine Learning Algorithms
At the heart of self-driving technology lie sophisticated machine learning algorithms. These algorithms utilize training data to achieve several objectives:
1. Perception
Perception algorithms are responsible for interpreting sensor data, understanding the environment, and identifying objects. They rely heavily on training data for:
- Recognizing traffic signals and signs.
- Detecting pedestrians and other vehicles.
2. Decision Making
Once the environment is understood, decision-making algorithms determine the vehicle's actions. Training data teaches these algorithms about:
- Optimal routes.
- When to accelerate, decelerate, or make a turn.
3. Control
Control algorithms execute the maneuvers necessary for the vehicle to navigate safely. Training data aids in:
- Adjusting steering angles.
- Modulating throttle and brake applications.
The Future Impact of Training Data
As technology advances, the role of training data for self driving cars will only expand. Innovations in AI and machine learning will necessitate:
- Real-time Data Collection: Harnessing cloud capabilities to analyze and adapt in real-time.
- Continuous Learning: Vehicles will learn from their experiences on the road, continuously improving over time.
- Global Data Sharing: Collaborations among manufacturers to share and combine datasets will enhance the training of more robust models.
Conclusion: The Path Ahead
The journey of self-driving cars relies heavily on robust and comprehensive training data for self driving cars. As these vehicles grow more sophisticated, the need for diverse, high-quality training data becomes increasingly critical. By addressing challenges associated with data quality, bias, and legal issues, we can unlock the full potential of self-driving technology.
In conclusion, the evolution of training data will not only dictate the reliability of autonomous vehicles but will also shape the future of transportation as a whole. As we stand on the cusp of a new era, embracing innovation and investing in quality data will pave the way for safer roads and smarter travel.